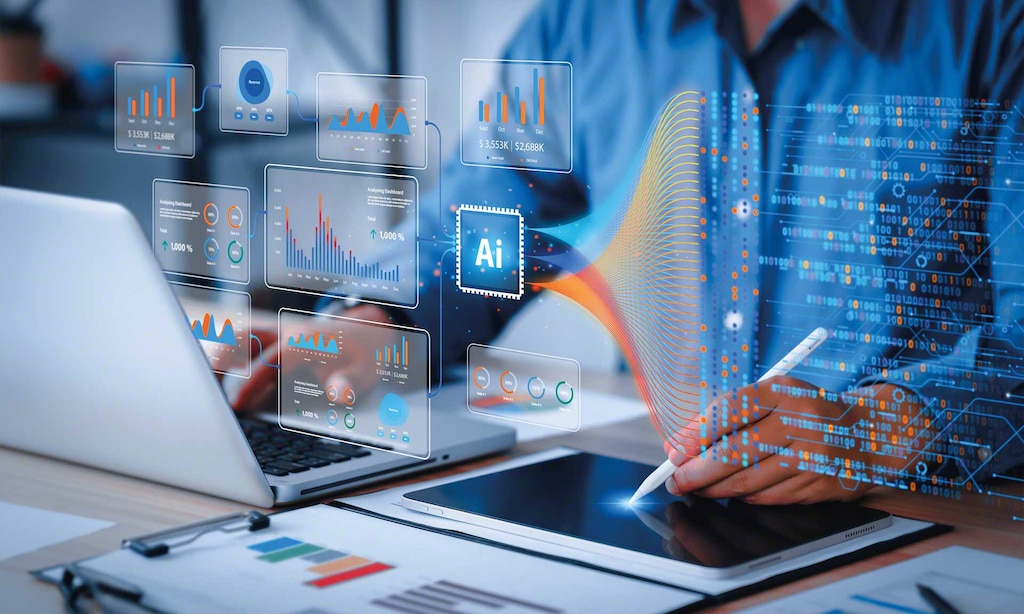
IN-DEPTH ANALYSIS
By Lawrence S. Maisel, Robert J. Zwerling and Jesper H. Sorensen
Executives Lawrence S. Maisel, Robert J. Zwerling and Jesper H. Sorensen argue that AI applied to data analytics presents an opportunity to create tools, techniques and business processes that provide insight into patterns, relationships and trends. In their book AI-enabled analytics for business: A roadmap for becoming an analytics powerhouse, they offer companies a guide for driving and implementing AI in corporate data analytics.
Artificial intelligence (AI) dates back over 75 years. Mathematician Alan Turing explored the mathematical possibility of AI, suggesting that “humans use available information as well as reason in order to solve problems and make decisions.” If this premise is true, then machines can do so too. This was the basis of his 1950 paper “Computing machinery and intelligence,” in which he discussed how to build intelligent machines and test their intelligence.
So, what is AI? Very broadly speaking, it is the ability of a machine to make decisions that are done by humans. But what does that mean, what does AI look like and how will it change our lives and society?
We all know that AI, sooner or later, will be part of all businesses. But when it is part of the business is entirely dependent on what each executive knows and understands about AI and analytics. And here lies the chasm between the early adopters and the rest of the pack.
Many executives lack clarity of vision as to how they will adopt AI in their business, division, group or department
Implementations of AI are just scratching the surface, as projects have been highly targeted to only certain areas of organisations and for certain tasks. So, while the movement to incorporate advanced analytics is in the right direction, there are many more failures than successes. The good news is that AI and analytics failures are eminently avoidable.
Many executives lack clarity of vision and voice as to how they will navigate their business, division, group or department through the adoption of analytics and AI. Other executives think they know what AI enablement means but are often working from poorly defined terms or misconceptions about analytics. Their knee-jerk response is to hire consultants and buy AI-enabled analytics software without fully understanding how analytics will be used to drive decisions.
Cries of “We need better forecasting,” “What factors are driving our business?” and “We must get smarter about what we do” echo in boardrooms and executive conference rooms. But how exactly is this done? The “what,” many an executive has read from a mountain of consulting reports, but the “how” is unclear and is why too many companies are lagging in their adoption of AI and analytics.
AI and ML: Similar but different
We see the widely used phrase “AI and ML” and conjure these as linked at the hip; however, while related, they are not one and the same. The overarching concept of AI is simply a machine that can make a human decision. Any mode of achieving this human decision by a machine is thus AI, and machine learning (ML) is one such mode or subset of AI. Therefore, all ML is AI, but not all AI is ML.
ML engages algorithms (mathematical models) that computers use to perform a specific task without explicit instructions, often relying on patterns and inference instead. Another popular form of AI is neural networks that are highly advanced and based on mirroring the synapse structure of the brain.
Machine learning primer
ML is a technique and technology that today requires specialised skills to use and deploy. ML is an AI engine often used with other tools to render the ML output useful for decisions. For example, suppose a bank wants to expand the number of loans without increasing the risk profile of its loan portfolio. ML can be used to make predictions regarding risk, and then the results are imported to spreadsheets to report those new additional loan applicants that can now be approved.
Large ML projects often involve the collaboration of data scientists, programmers, database administrators and application developers (to render a deliverable outcome). Further, ML needs large volumes of high-quality data to “train” the ML model, and it is this data requirement that causes 8 of 10 ML and AI projects to stall.
While ML is popular and powerful, it is not easy. Many new software applications are making ML use simpler, but it is still mostly for data scientists. Before an ML project can begin, its “object” must be defined: that is, what is to be solved. For example, suppose we want to predict which customers on our e-commerce website will proceed to check out (vs. those who exit before checking out). The process to go from the object to the deployed solution has many steps, including data collection and preparation, selection of the algorithm and its programming, model training, model testing and deployment. Any failure at any point will require a reset and/or restart back to any previous point in the process.
While complex, machine learning can offer high business value with a wide range of applications
ML has a limitation in that the solution of the object is highly specific to the data used to train the ML model. Most often, the model is not transportable, even to a similar company or a similar department within the same organisation. Also, as mentioned, the use of ML often requires other tools to render its results advantageous for consumption by business managers.
However, while complex, ML can offer high business value with a wide range of applications: for example, predicting customer churn, sales deals that will close in the next 60 days, drugs with a high probability of advancing to the next phase in trials, customers who are more likely to buy with a 5% discount, demand forecasting, and so on.
We live in an exciting time for change. Much has been done by business to advance productivity, and with it, people’s lives. For example, at the turn of the twentieth century, the invention of electric power and the electric motor fundamentally and dramatically changed society, with immense benefits for mankind. Even more than the electric motor’s introduction, AI will make profound changes over the next generation and beyond.