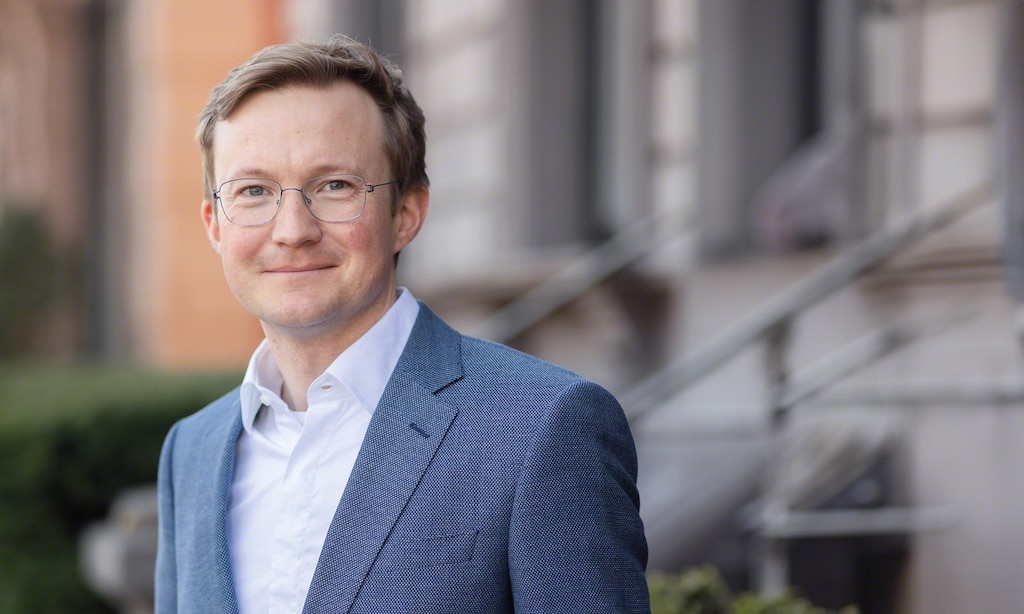
“High-resolution, near-term demand prediction is a game changer for e-commerce businesses”
Watch the video of the full interview >
Matthias Winkenbach, Principal Research Scientist at the Massachusetts Institute of Technology (MIT)
About the expertMatthias Winkenbach is a Principal Research Scientist at the Massachusetts Institute of Technology (MIT) Center for Transportation & Logistics. He founded the MIT Computational Analytics, Visualisation & Education (CAVE) Lab, where he leads a team of researchers, UI/UX designers and software developers to create technologies for visual supply chain analytics applications. Winkenbach has worked with numerous global industry partners to solve problems that have real-world impact on people, profits and the planet. He regularly advises start-ups and established industry players on projects involving supply chain analytics, logistics systems design and last-mile technologies.
Mecalux interviews Matthias Winkenbach, Principal Research Scientist at the Massachusetts Institute of Technology (MIT), to analyse the AI trends set to have a significant impact on the future of e-commerce.
You recently said that AI will be the next big thing in the supply chain. What’s its potential to improve logistics?
There are many ways in which artificial intelligence (AI) could have potential in the logistics industry. The part that my team mostly looks at is on the systems’ design level. So, how AI will enable industry to incorporate not only more data but also richer datasets when solving problems. Think of a straightforward example like route planning. It traditionally has been driven by operations research algorithms that have a simple goal: finding the shortest, cheapest and fastest route. In real life, however, a company’s objectives are much more complex. You want a route to be cost-efficient but also safe and follow other intricate guidelines that are impossible to address with traditional algorithms. Machine learning models can solve these issues — they can incorporate more realistic objective functions and data to tackle logistics challenges like inventory management, network design, road conditions or customer-specific needs.
How are you testing these technologies at MIT?
I lead two labs. One is the Megacity Logistics Lab, where we conduct research on last-mile logistics, urban mobility, e-commerce distribution and omnichannel network design. The other one is the CAVE Lab, which is focused on software development: we build tools for our research partners to work with analytical models and large-scale logistics data. We design human-centric interfaces to interact with these models in a hands-on way, so you don’t need to be a technical expert to use them.
What key challenges do e-commerce businesses face when optimising their distribution processes?
The e-commerce world has changed quite a bit over the last couple of years. When I started my research in this space, we were in a world where you would order much less online and expect it to arrive within four to five days. Now, the expectation is to get it the next day — and I’m pretty sure in the near future it will probably be same-day or sub-same-day delivery. This immense increase in speed and the tremendous growth of the assortment of products you can order online are adding layers of complexity and challenges that the logistics industry has to somehow solve. One is, for instance, inventory management and positioning. In a longer-delivery-lead-time world, you wouldn’t need to worry about inventory because you can reach 80% of the US population within two to three days from pretty much three distribution centres spread across the country. Now, in a next-day world, you need at least nine or 10 facilities across the country to reach the same number of people within that time.
What about a same-day delivery world?
As we’re strongly moving towards on-demand e-commerce, where you order a product and get it within an hour or two, a centralised system just doesn’t work any more. You have to be more local; you need a more fragmented network of facilities. And that also means all your inventory gets fragmented. You must be smart about what kind of product and in what quantity you store where. Otherwise, you’re going to duplicate stock all over the place — and that’s extremely costly. Inventory management and network design are two of the biggest hurdles e-commerce businesses face. Related to them are challenges like demand prediction: if I can predict more accurately not just what my demand for the next month is, but what my sales in lower Manhattan within the next two hours will be, then that’s a huge game changer. With more granular, near-term demand prediction, companies can reduce their overall inventory requirements to offer a faster and more reliable service to their customers.
The immense increases in speed and online product variety are adding challenges that the logistics industry has to solve
How can AI help address e-commerce distribution challenges?
AI may help in many ways. Besides improving routing, another example is network design and inventory planning. Recently at the lab, we’ve been working with a retailer who wanted to design a last-mile distribution system that enables a superior omnichannel experience. Think of having a certain customer group that’s very influential to your business. Let’s say you’re selling fashion and you want to reach the influencers of this world with a premium service like ordering online and getting the product within 60 minutes, regardless of where they are in a given city. Designing such a system is impossible with traditional software because it’s too complex. There are too many variables that are suddenly no longer following linear relationships and the computational effort of figuring all of this out gets too huge to solve in reasonable amounts of time.
That’s where we leverage AI algorithms to make better decisions about where to place inventory, which physical stores the company should use for this service or where to establish additional facilities that only serve the online channel. We’re seeing how machine learning and operations research are becoming more equal partners in solving these very complex logistics problems.
What can businesses do to be the first in shipping times?
To prepare for this race to the bottom in last-mile logistics, I think organisations need to keep in mind that it all boils down to being able to design networks that are made for this kind of service, not just retrofitted. Customers are expecting different delivery times and portfolios of products to be purchased online and companies are trying to make it work out of their legacy infrastructure. However, if you want to do it right, sometimes you have to take the leap and redesign things from scratch. Obviously, you want to leverage the infrastructure that still makes sense. But, for instance, if you have a warehouse 50 km outside the city and you want to offer two-hour delivery, that’s just not going to work. You need to invest in infrastructure that’s dedicated to these high-speed services.
The other crucial aspect is that you have to invest in analytics. Many logistics companies have historically been focusing on optimisation problems. They usually have specialised teams that work on the engineering side of building and operating logistics networks. However, there are not that many businesses with a dedicated data science team. The race to this supreme level of service is also a race for talent and finding the right experts who can build the next generation of algorithms your company may need. This is because the skill set of these professionals is slightly different from the engineers that you used to hire 10 to 15 years ago. You also want to invest in people who can translate between the logistics side of things — the business perspective on the problem — and individuals with a more technical perspective.
How critical is it to build a bridge between these two worlds?
Some organisations make the mistake of just hiring a bunch of data science PhDs who’ve never worked in the logistics industry before. Even though they’re very competent, there’s usually a divide between those experts and the people who know how the business works. We see many companies struggle with that. I think the key is to hire people with a mixed set of experience. If we look at industrial engineering programmes here at MIT or elsewhere, we’re already trying to educate students in this direction: you still need a solid industry understanding and a good engineering background, but you also require a deep enough knowledge of data science and machine learning. You don’t have to build the latest incredible machine model, but you need to be able to talk to your data scientist who built it for you and to the logistics manager who knows exactly what he or she is doing even if he or she can’t write a single line of code.
What emerging AI technologies or trends do you believe will have a significant impact on the future of e-commerce distribution?
It sounds a bit old right now because everybody is talking about chatbot technologies, but I think these types of methods will have a huge impact on the industry. One way and that might surprise you, is that the same type of methods that power tools such as ChatGPT are very promising in solving some of the logistics planning and optimisation problems that we’ve been trying to tackle for many years. Methods that are relatively similar to the ones that power these chatbots could be very useful in solving problems such as predicting a good route rather than optimising it. And that’s going to affect not only routing but also inventory and network optimisation. Pretty much every big combinatorial optimisation problem out there could eventually be tackled with these kinds of methods.
On the consumer side, the main revolution that we’ll probably see is seamless interaction with e-commerce platforms. You’ll no longer need to physically go onto a website, find a product and click buy. You’ll just talk to your AI agent on your smartphone and say: “I forgot to buy toothpaste; can you get me some?” And then it will probably analyse your previous purchasing behaviour to understand which brand you usually buy and what your acceptable price point is. I think the entire e-commerce experience will become much more fluid and integrated into everyday life, which also means, by the way, that this will push the trend toward on-demand consumption even further. That also implies that the logistics systems behind this will need to become much more adaptive and dynamic in being able to respond to this. Because if you have this fluid interaction, on the one hand, but then you have to wait four days to get your stuff, that kind of defeats the purpose. So, I think that’s probably where the e-commerce world is heading, maybe not today or tomorrow but in the medium term.
The challenge is how to get products closer to customers in a more sustainable way
What advice would you give to e-commerce companies looking to adopt AI in their distribution processes?
Now that these chatbots are out there, people suddenly realise what these models can do. But AI is still a black box. Sometimes, when I talk to industry representatives, they tell me they want to invest heavily in AI because it will solve all their problems automatically. However, these methods are still relatively nascent. They have huge potential, but we need to understand them better. It’s not like you can just pick a model off the shelf, throw it at a problem and it will fix it. AI is not the holy grail; it won’t solve every single challenge immediately with no effort.
If you want to dive into this space as a company, a researcher or whatever your role might be, start small. Identify issues that you understand very well already. Don’t pick problems that you have no clue how to fix. Pick those that you know how to solve, like routing or inventory management and try to fix them with a machine-learning approach. Once you figure that out, you can always add complexity and additional topics. If your resources are limited, then try to invest your money smartly in simple-to-solve issues to gain experience and build talent. I think that’s how you can grow an analytics capability within your company that can solve problems, learn from them and merge these learnings over to the next big challenge. Don’t think that you can just build an AI model, throw your entire supply chain at it and it will fix it miraculously. That’s not going to happen.
How do you see the future of last-mile e-commerce distribution?
I think last-mile distribution is going to become even more individualised to the extent that consumers may be more and more in a position to tailor the delivery experience to what they need and where and when they need it. In the future, this whole concept of getting home delivery might become more fluid. Maybe home is no longer just your physical address but where you are at a certain time of the day. You may no longer need to specify whether you want your delivery to your home address if the algorithm can tap into where you are and drop it off there. I’m not sure if that’s always desirable, but at least that option will be there to have a more dynamic approach to when and where to receive shipments.
That goes hand in hand with a general trend towards faster and more individualised, more on-demand delivery approaches. And quite frankly, I think e-commerce will also take over a much larger part of the overall retail market. It’s already grown quite rapidly over the last couple of years and it will continue to do so. The advent of AI and other technologies makes the online buying experience increasingly close to physically making purchases in a retail shop.
How is this experience shifting?
Many people still go to shops for certain products because they can’t get them at the same level of quality or immersiveness online in front of a screen, but that’s changing. Think of all these new fancy technologies around augmented reality. There’s a lot to come there that might have lasting effects on how we shop for certain products. For the logistics industry, that just means that business won’t go away. If at all, there will be more business. But building the systems and the processes to be able to keep up with all these customer requirements is going to be a challenge. Companies will have to think about the next generation of logistics systems that they want to build because in an increasingly on-demand world, the legacy systems that you built 10 or 15 years ago at some point will just no longer work. So it’s better to invest in this early than to be surprised at a very late stage of the game.
An increasingly on-demand world can make sustainability more challenging.
The e-commerce industry is seeing criticism that with people buying more and more products online, everything gets shipped to the door and the immediate conclusion is that it can’t be sustainable. Honestly, it’s not that clear that moving to a next-day or same-day delivery world would necessarily be a huge problem when it comes to emissions caused by e-commerce — as long as these systems are managed and planned well and the companies around them have strong analytical capabilities. To give you an example, if I order a pair of running shoes online and I want them in two hours, that means they already need to be somewhere close to me to be delivered fast. It also means that the product is close enough that it can be shipped with an electric vehicle or a cargo bicycle.
This need for proximity opens up the opportunity to sustainably power last-mile logistics fleets, which you could not currently decarbonise if you had highly centralised inventories far outside the big cities. The challenge is how to get the package close enough to you in a more sustainable way. Do you have the right analytical tools to help you anticipate where you need that pair of running shoes so that you don’t store them far away from the customer on the other side of the city? You have to be able to predict well to avoid storing products in the wrong places. Likewise, you must be equipped with a system that allows you to consolidate the replenishments of these localised inventory locations that eventually serve you with your running shoes. And you want those facilities to be replenished in a consolidated matter, with a low-emission vehicle in an efficient way. This is all possible, but it needs to be planned and designed properly.
AI could become crucial in sustainable logistics.
If companies respond to this move toward more speed and on-demand consumption wrongly or poorly, it will lead to a massive increase in emissions. But if done properly, it can enable a much more effective decarbonisation of the distribution process because it moves fulfilment closer to the consumer. AI can play a crucial role in sustainability in the future of logistics. If we’re serious about decarbonising freight, I think you’ll need the analytical power that will probably only come from integrating AI and machine learning more strongly, more deeply, into the logistics planning and execution processes.