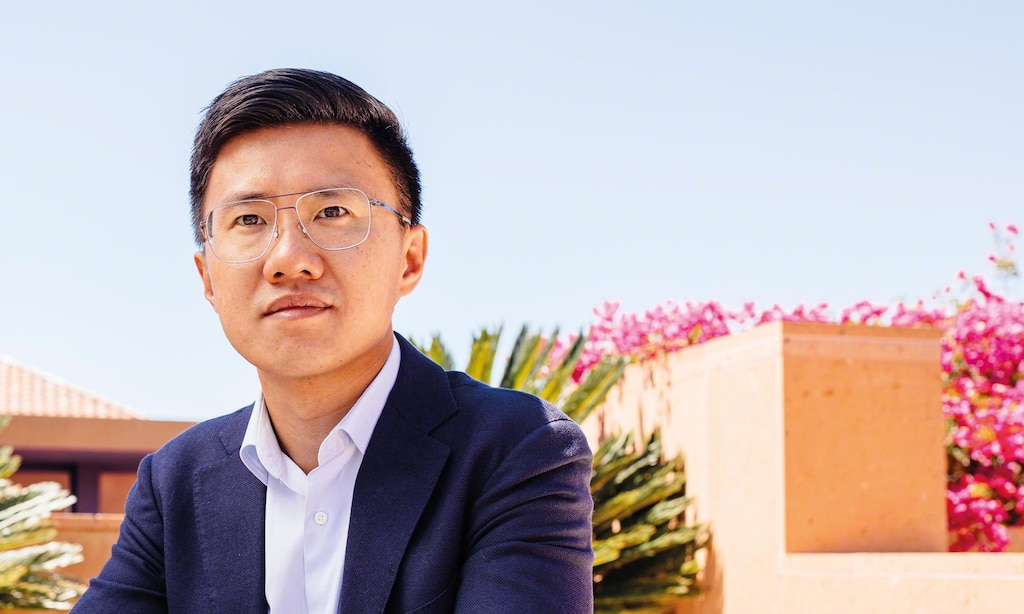
“If you’re a company in the logistics space, you absolutely should think about integrating AI”
Watch the video of the full interview >
Prof. Kuang Xu, Associate Professor of Operations and Technology, Stanford Graduate School of Business
Kuang Xu is a tenured Associate Professor at the Stanford Graduate School of Business. He is an expert in operations research, AI, data science innovation, supply chains and logistics and data-driven decision-making. He is a co-creator of AI and Data Science Strategy, wich is the first Stanford course focusing on the strategy, management and entrepreneurship of AI and data science. Xu is a Co-Director of the Stanford GSB Value Chain Innovation Initiative.
Professor Xu teaches Executive Workshop on AI and Data Science for managers, founders and business leaders around the world. It covers how to leverage AI and data science to power business and operations, how to innovate in data science and AI and integrate them into existing operational flows at scale and the best practices in developing ML/AI-heavy products and work processes. You can reach out to Prof. Xu at kuangxu@stanford.edu.
As an Associate Professor of Operations and Technology at the Stanford Graduate School of Business, Prof. Kuang Xu is a firm believer in the transformative power of artificial intelligence (AI) in business. In this interview, he shares his perspectives on why companies should embrace automation and data-driven decisions to unlock AI’s full potential.
-
Your research at Stanford focuses on decision-making under uncertainty. What would you recommend organisations invest in before the next decade?
Everybody is thinking about generative AI such as chatbots for customer service and the like, but the business case hasn’t been proven quite yet. We’re still in the phase of assessing the business value of these kinds of headline-AI type of agents. So, instead, I thought I might bring up a few observations on the types of technologies that we know deliver value today.
Machine learning and AI grab a lot of attention. You can think of this at the high level as two pieces. The first one is getting information and making predictions — basically, asking the question as what the world is. The second piece is what I call optimisation and decision-making, that is: Given the world is this way, what should I do? The first piece is easier to understand, more intuitive. The second one is more difficult, less interpretable. However, it’s a really critical part. Knowing “what the world is” is important, but then acting upon it is what ultimately delivers value.
-
So, businesses should allocate funds to both?
One big part companies will continue to benefit from may not come as a surprise: invest in good data-driven optimisation for your logistics processes and your supply chain. Consider important aspects such as how you route, where you place goods and where your facilities are. Collect the data, make predictions and have the capability to use these in an optimisation engine to change your allocation, routing or logistic network. In summary, develop good AI- and data-driven optimisation models.
The second aspect is less talked about, but it’s the “glue” in the logistic value chain when it comes to AI and machine learning. To clarify, when logistics and supply chains are designed by humans, humans are the glue. For example, when you send a shipment or a data file, if there’s an error or ambiguity, we call each other up and sort it out. It does cost money and friction, but you don’t think of this as a special capability. What we’re seeing now, though, is that it’s often easy to isolate one task and then have a machine do a little better in some way or form. Most of the time it can probably tell you where to send the lorries and which shelf to go to and optimise. However, when it breaks, you have to engage a separate backup system that often involves humans. These technologies grab headlines saying, “Wow, 95% of the time it would do better than a human in X.” But it turns out that integration between that 95% and the 5% when they cannot is really tricky. So, why am I harping on this so hard? Because the glues are so important.
AI’s potential in optimising physical processes such as supply chain logistics is increasingly evident
-
How so?
You would think, OK, without the glue, the system still works. No, it does not. Not having the glue doesn’t mean you just get 80% of the 95%. It means you often get nothing. It just prevents people from making the whole system work. In fact, in this class we’re teaching at the GSB, we look at historical examples of companies in logistics and healthcare that use machine learning and AI. And a very coherent theme is that many times, the secret sauce of success is not a better machine learning algorithm. The winners tend to be the gluers. Why? Because they’re good at both developing technology and investing in the product design to make that integration much better than their competitors. So, I would just say: invest in AI integration. It’s probably less talked about but super important.
-
You advise companies and investment funds on how to build core AI and data science capabilities. What are your key recommendations?
It’s a very case-by-case type of advice. If I were to summarise one key aspect, it would be that oftentimes, before businesses jump into the technology, what they truly miss is not identifying the most accurate machine learning technique, but framing and defining the value of the product they are delivering. The question you need to ask is: “If I had the technology, how much value would that really drive?” And I don’t mean value in a very abstract sense such as branding or company culture, which are also important. I’m talking about something a bit more concrete: Can you literally write down a metric and connect it to the accuracy and statistical performance of your technology? If so, does that save you money?
Surprisingly, that’s not an exercise a lot of people do. What’s much more common is they believe the technology will bring some general capability and they just go in for it. They’re not really sure what success looks like. Maybe they assume that the technology will replace an entire existing product line or drastically enhance productivity, but it’s never clear in their head whether these expectations are really feasible. Therefore, you may get into the complication where investments might be made without tangible benefits. I think defining that value early on with someone — your team — who understands the capability of technology and the potential of machine learning for your business is crucial.
-
“Cost savings are a big part, although the hope is for AI to open up entirely new product offerings” Are organisations implementing AI in different areas out of the fear of being left behind?
Absolutely, that’s happening. The way I like to frame it is: data-driven decision-making is as much of a science as it is emotion and psychology. I don’t mean that in a way that it’s something to be avoided; rather, it should be embraced. But one has to know that one is fearful. I see a lot of companies facing pressure from shareholders, stakeholders and boards to demonstrate they’re actively investing in AI. So, the fear isn’t entirely unwarranted.
I think there will be substantial seismic changes in the industry because of not just potentially step-changing AI, but the consistent sustained growth in AI and machine learning over the last decade. So yes, I do think companies need to be fearful in the sense of: “If you’re entirely unexposed to this, you do have the chance of being left behind.” However, I think the way to engage it is to embrace that fear, make sure you know what’s going on and ask, in the short term, what value can you hope to get out of it. I also see a lot of businesses jumping in and investing hugely in generative AI, bringing on new leadership…. People joke that these days, the hottest job is a director of AI in some conventional company. You need to be very careful. A better approach is: yes, embrace that fear. This thing is real; it’s definitely happening. But exactly where it’s happening in your organisation is not so cookie-cutter. Not everybody is going to benefit from an automated chatbot. So, think about value. That will guide you better.
-
What businesses should really adopt AI?
That’s a fantastic question. If you’re a company, especially in the logistics space, where your operations are already automated or have the potential to be automated with telemetry — with feedback and measurements — then you absolutely should think about integrating AI. Many organisations are already using manufacturing robots and logistics warehousing solutions like your company is doing. The reason is automation is the gateway. Without automation, without feedback, these techniques won’t have the stage to perform.
But once the gateway is open, competition will be up. Because sooner or later, others will automate better than you do. So, that’s definitely an area you want to look into. Any time the physical world connects to the internet and databases, AI will come in. While AI’s roots lie in manipulating digital information, its potential in optimising physical processes such as supply chain logistics and transport is increasingly evident. Companies like DoorDash, Uber and Lyft are the first wave. They’ve integrated drivers into an app, gaining a degree of control over optimisation in the physical world. If you take that analogy further, you might wonder: What else has already been integrated or has the potential to be integrated? Automation and integration into the net are key domains where AI is poised to make significant strides.
-
What other fields hold promise for AI?
The second area I would emphasise is: How much does your operation depend on information? Let’s say the data indicated a different demand pattern, a different cost structure and a different supply chain flow. Would that information have drastically changed your decision for your business? Let’s take an example in real estate, where things work on a larger time scale. You buy a building and rent it out. If there’s fluctuation in the city’s population flow today, you’re not just going to sell the building right away. Even though the possibility of using AI obviously exists and it may help, your decision is less sensitive to the flood of new information, so you can also just take your time to analyse the scenario. However, in a company where your day-to-day operations depend so crucially on the information available at that time, AI can really make an impact. Real-time delivery is a great example because you need to react to the market and environmental conditions quickly. In these businesses, having better information access can help you change your operations dramatically.
Another way to say it is: You want an environment where, number one, there’s uncertainty, so the context changes and new challenges come up. And two, the uncertainty affects your business decisions. That’s an area that’s very ripe for AI and it will help you. If neither is true, if your environment is not changing at all — or if it does, it has no impact on your company — then the margin will likely be less.
-
Beyond cost savings, what unique opportunities can AI enable in businesses?
Cost savings are a big part, although the hope is for AI to not just reduce costs but open up entirely new product offerings. You can always think of the application where AI replaces workers and people may be concerned about that. However, you can also think of examples where AI offers new experiences that weren’t available before. There are emerging applications where the experience is only possible with AI and that could be a great opportunity to expand.
In logistics, what’s very interesting — and I’m glad to be proved wrong — is that everything we see is very much compressed to a cost. It doesn’t matter where you get your screwdrivers as long as their quality is good and the cost is competitive. Now, could there be a scenario where how you get a screwdriver or the reliability of your warehouse delivers a completely different experience for a customer? That would be very interesting to look out for. You may want to ask: Once I put the robots in, what if it’s not just cheaper to retrieve a screwdriver, but also much more reliable? What if that allows me to get data that enables me to package it into a new product? When AI empowers you to do something you simply couldn’t do before, I think that’s a fantastic area to look into.
-
You once said in relation to AI that the goal is to have a tool, not a lord…
This idea is connected to the fear. Fear is a natural emotion — everybody has it. The goal is not to avoid experiencing it but to be aware of it and leverage that energy to your benefit. I think fear is an indicator that something is happening and you should pay attention. It’s an alert mechanism to bring awareness. However, if you don’t consciously acknowledge it and the fear gets really deep, it starts to dictate what you do. You stop thinking. That’s something that we definitely see a lot; the fear of missing out causes you to freeze in some way.
Another way to think about the concept of AI as a tool versus a lord is that a lord defines the objective for you. It tells you, “I’m this technology, this is what I do, maybe you should do it too.” That’s an example where you copy-paste a solution without thinking deeply about what value it drives for you. Whereas a tool doesn't define value — it executes an action and you’re the one who’s supposed to define that value. So, if you think of AI as a tool, the question you really want to ask is: Do you get to define and understand the value you’re trying to achieve and did you do your homework to get there?
-
"In logistics, the key is to design algorithms that quickly adapt to a highly non-stationary environment" What are your latest findings on AI-powered analytics to help companies make better decisions?
One research area we’re exploring is sequential learning — also known as reinforcement learning — where an AI agent is trying to act optimally in a world but needs to interact with it first in order to learn. When you interact with the world, your actions have consequences. This affects how much information you collect, where you collect it and your experience at the time. You don’t want to send a robot into the middle of the street to gather information only to be run over by a lorry. We’re studying how to design that robot to collect information and act while learning.
A new area that we now realise we haven’t quite understood yet is how to perform this type of task in an environment that doesn’t stay still, which — if you think about it — is almost all we interact with. Whenever we come to a new environment, at the beginning, there’s always a learning process, but as you learn, your surroundings might change as well. So, how do you design agents that are robust and can seek out the correct information in these changing environments?
We’re quite active in this area at Stanford GSB and it’s exciting because it enables you to tackle many applications that we previously addressed using an approximation, where we pretended the environment wasn’t changing. If you think about online recommendations or online retail, these changes are the norm. Agents that are currently being deployed for companies that use AI to learn to price and recommend are still designed for a stationary environment. We already see big gains when you use some of our findings in those areas. When I’ve worked on projects for clients in logistics, this is a crazy, highly non-stationary environment — you have regulation, disruptions, produce seasons…. Here, the key is how you design algorithms to quickly adapt and get the right information with AI.
-
What role do you think AI will play in the future of logistics?
It’s hard to say, but the big-picture question here is: Why is AI making so much noise in chatbots and image generation and not so much in logistics? I think the main reason is quite simple. Moving physical goods is hard in many aspects and also difficult to get a margin on. The question is how to reduce friction at every point along the path. You have to think about a container ship and an AI, analyse how many steps are in the way and figure out how to reduce the friction along the chain. For instance, maybe with AI, there’s a better method to communicate a certain decision on which dock the lorry should pull up to at the warehouse.
Instead of thinking about AI, I recommend companies first think about where they can reduce friction — that’s currently a bottleneck. Once that friction is low, there are so many ways AI can make an impact. Right now, I believe friction is the biggest bottleneck. Some businesses that are successful are good at solving that bottleneck. Organisations are not successful because they have the weirdest, most amazing AI team — they probably do have a good AI team, but that’s not the critical part. The critical part is the platform, the operations: getting the operations to the point where computers can move atoms. That’s the big-picture obstacle right now. But I’m excited. The robotics you’re building at Mecalux could be a great step towards that. Overall, if companies can use more technology — sometimes not even AI — to connect things and reduce friction, then we’re talking. I’m very optimistic about the future of AI in warehousing and logistics. It will absolutely keep growing.