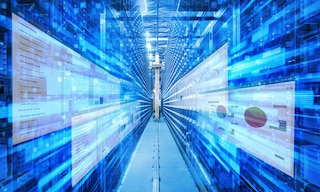
Data mining in Logistics 4.0
Data mining is the analysis of large volumes of data to identify patterns and trends that reveal valuable information to support decision-making in organizations.
Data mining can help companies to understand the behavior of their processes and operations ― including those relating to logistics ― and to make decisions that improve their performance.
What’s data mining?
Data mining encompasses the computational process of identifying trends, rules, hidden patterns, and other valuable information through the analysis of large sets of data. Also known as knowledge discovery in databases (KDD), data mining has become much more relevant in recent years due to the increase in data storage technologies (big data), artificial intelligence (AI), and robotic process automation.
Among non-experts, the term data mining is often confused with big data technology. Both terms refer to related but ultimately distinct concepts. Big data refers to data sets so massive and complex that they require software applications to process them. Data mining goes a step beyond: it involves reviewing that extremely large volume of data to detect rules and patterns that are hidden to the naked eye.
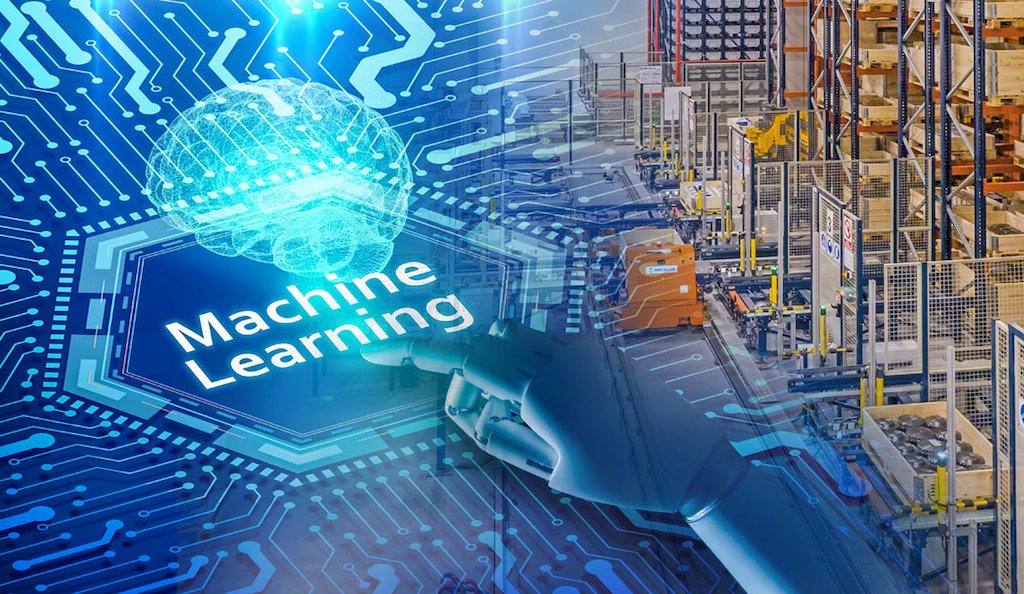
To grasp how data mining works, it’s essential to understand the relationship between this analysis method and technologies such as AI and machine learning. AI and machine learning systems use data mining techniques to interpret machine behavior and create solutions from the patterns and rules identified in the data. In fact, as indicated in the publication Algorithm Insights from consulting company Deloitte, data mining belongs to the category of cognitive technologies, i.e., those that facilitate the implementation of AI systems (which includes machine learning).
Data mining constitutes the process of analyzing and extracting hidden and actionable knowledge from large data sources stored in different formats. In his book Data Mining: Practical Machine Learning Tools and Techniques, Emeritus Professor of Computer Science Ian Witten says: “Data mining is the extraction of implicit, previously unknown, and potentially useful information from data. The idea is to build computer programs that sift through databases automatically, seeking regularities or patterns. Strong patterns, if found, will likely generalize to make accurate predictions on future data.”
Data mining enables better decision-making in all areas of an organization. Automatic data extraction methods make it possible to organize and filter information to transform it into relevant knowledge that helps in certain areas to detect fraud (finance), predict demand (sales and marketing), and identify bottlenecks (industry and logistics), among many other applications.
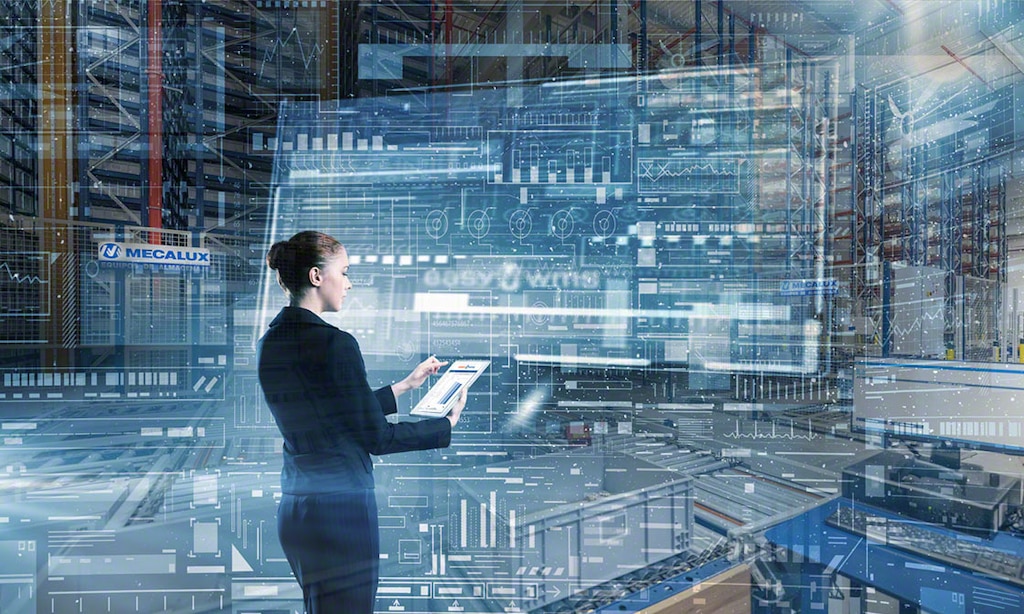
Applications of data mining in logistics
Logistics could be one of the areas that benefits the most from the consolidation of data mining. The automatic detection of patterns in operations such as goods receipt, order picking, and product returns could enhance stock demand forecasting and inventory control.
In his academic paper A Review of Supply Chain Data Mining Publications, Supply Chain Management and Analytics Professor David L. Olson of the University of Nebraska says that data mining in the supply chain is already a reality: “Supply chain operations have been supported by the usual business data mining analyses of classification (to include customer profiling and fraud detection) and prediction. That implies use of the standard data mining methodologies of logistic regression, decision trees, and neural networks for classification, and regression for prediction.”
The author says that the impact of data mining in logistics will increase: “The application of this computer technology to generate measurements of important aspects of supply chains, and to analyze this data to make better decisions, will continue to grow.”
Beyond decision-making in inventory management, data mining could ramp up throughput in logistics phases such as freight consolidation. This is reflected by researchers Bruno Agard and Zineb Aboutalib of Canada’s Polytechnique Montréal engineering school. In their analysis Improvement of Freight Consolidation with a Data Mining Technique, the authors say: “The implementation of association rules into the development of consolidation strategies permits the number of deliveries to decrease. This method in turn could also increase the quantity delivered in the same vehicle or delivered to the same destination, thus reducing transportation costs and carbon footprint.”
Data mining: in pursuit of logistics efficiency
Data control, processing, and management are key for identifying errors and inefficiencies in logistics and production centres. Data mining techniques facilitate the processing of the thousands of data produced in a smart warehouse, identifying trends that are hidden from plain sight. This information enables logistics managers to make more accurate and more informed decisions based on actual warehouse performance.
In logistics, where it’s becoming more and more common to monitor throughput using the data generated in the facility, a warehouse management software program such as Easy WMS plays a vital role. Interested in transforming the huge amount of data produced in your facility into valuable information? Don’t hesitate to contact us. One of our expert consultants will work with you to come up with the best digital solution for your warehouse.