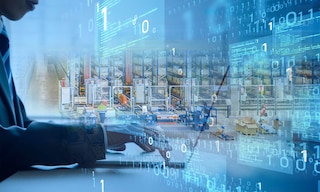
Small data: definition, differences with big data and application in logistics
In the information age, data play a fundamental role in corporate development and success. Although the concept of big data has been widely adopted by companies, there is another equally relevant but less well-known term: small data.
In logistics, small data provides multiple applications that contribute to improving efficiency, service quality and customer satisfaction. By focusing on specific, detailed information, companies can make better decisions, quickly adapt to market demands and achieve a more favourable competitive position.
What is small data?
Also known as microdata, small data refers to a data set more limited in size and scope than big data, which describes a data combination known as the three Vs: volume, velocity and variety. Small data is characterised by being more manageable and highly focused, and encompassing specific information.
The small data concept, popularised by marketing expert Martin Lindstrom in his book Small Data: The Tiny Clues that Uncover Huge Trends, came about as a response to the exponential growth of data in the digital world. As the amount of available data increased, so did the complexity of handling them and transforming them into useful information. In this context, small data presents itself as an alternative way of obtaining relevant and valuable information without having to address the big data challenge.
According to Lindstrom, small data can provide deep insights into human behaviour and consumer preferences, allowing companies to make more informed decisions and develop more effective strategies to meet market demands. For Lindstrom, small data is based on the idea that not everything can be understood through huge data sets and mass analyses. Sometimes the key to unravelling customer buying habits, emotions and needs lies in looking at the smallest yet most significant details of their everyday lives: “A lone piece of small data is almost never meaningful enough to build a case or create a hypothesis, but blended with other insights and observations gathered from around the world, the data eventually comes together to create a solution that forms the foundation of a future brand or business,” Lindstrom says.
Differences between small data and big data
The differences between small data and big data are appreciable and mainly based on volume, focus and the way data sets are processed and analysed.
- Data volume. Small data handles far smaller data sets, so it can be processed and analysed using simpler resources and tools. On the other hand, big data works with mass data sets that require special technologies and tools to efficiently store, process and analyse all the information.
- Focus and relevance. Small data concentrates on specific and detailed information with a more focused and precise approach. It sifts out information that is extremely relevant for addressing specific problems or needs, which enables faster decision-making. The focus of big data is usually broad and covers a wide range of information that is often diverse and not always relevant to a specific objective.
- Tools and resources. Small data can be processed and analysed using tools that are both more accessible and less expensive: companies can use standard data analysis software to transform the information into smaller data sets. On the contrary, analysing big data requires technological infrastructures and advanced tools due to the enormous volume of information and the need to process it quickly.
- Applications and objectives. Small data is used to obtain more specific and focused information to better understand customers, personalise experiences, optimise processes and improve decision-making in situations that don’t require the analysis of large amounts of data. Big data, meanwhile, serves to identify patterns and trends in massive data sets, which enables strategic decision-making, predictive analytics and improved operational efficiency in areas such as marketing and finance, among others.
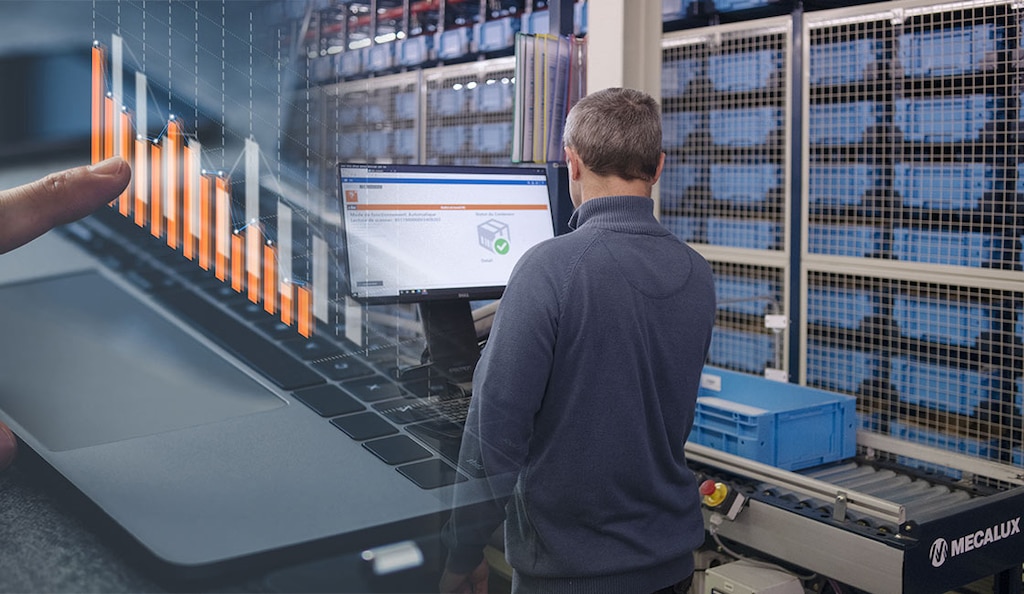
The benefits of small data
Small data offers a number of significant benefits, particularly in contexts where large data sets are neither necessary nor practical.
- Faster, easier decision-making. Smaller data sets can be quickly processed and analysed, facilitating speedier and simpler decision-making.
- Customer centricity. Small data enables a more detailed and personalised analysis of customer details. By better understanding their individual preferences and needs, companies can offer more tailored products and services, thus improving customer experience and satisfaction.
- Resource optimisation. Businesses can save resources in terms of time and costs because they don’t need to invest in complex and expensive infrastructure to process large volumes of data.
- Higher data quality. By concentrating on the specific and relevant details, small data tends to be more reliable and accurate. Information can be collected from select, verified sources, thereby lowering the likelihood of errors and redundancy.
- Identification of business opportunities. By focusing on specific details, companies can discover new market niches, emerging trends and areas for improvement that make a difference to their business goals.
- Privacy and data security. Organisations can mitigate the risks associated with privacy and security since cutting down on the amount of information stored makes it easier to implement data protection measures.
Small data applications in logistics
The use of small data in the logistics sector offers multiple applications and benefits that improve efficiency, decision-making and customer satisfaction.
- Optimisation of routes and deliveries. Analysing traffic data, weather conditions and delivery schedules optimises transport routes. By using specific and detailed information, companies can improve their logistics planning, shorten lead times and limit shipping costs.
- Inventory management. Real-time inventory data tracking and analysis prevent shortages and excess stock. Small data helps maintain a more accurate inventory monitoring process, thus reducing storage costs and preventing losses due to obsolete or expired products.
- Demand forecasting. By analysing historical sales logs and purchasing trends, small data helps predict future product demand. Businesses can then adjust their inventory levels and meet demand effectively, avoiding losses due to stockouts or overstocking.
- Improved customer experience. Small data facilitates the collection and analysis of information on customer preferences and behaviours to personalise the shopping experience and offer products and services more appropriate to consumer needs.
- Analysis of logistics costs. Small data simplifies the analysis of the costs associated with different aspects of the supply chain, such as transport, storage and material handling. By obtaining detailed information about costs, companies can identify areas for improvement and avoid unnecessary expenses.
- Detection of anomalies. By analysing throughput data and logistics operations, small data makes it possible to detect anomalies and potential problems in the supply chain.
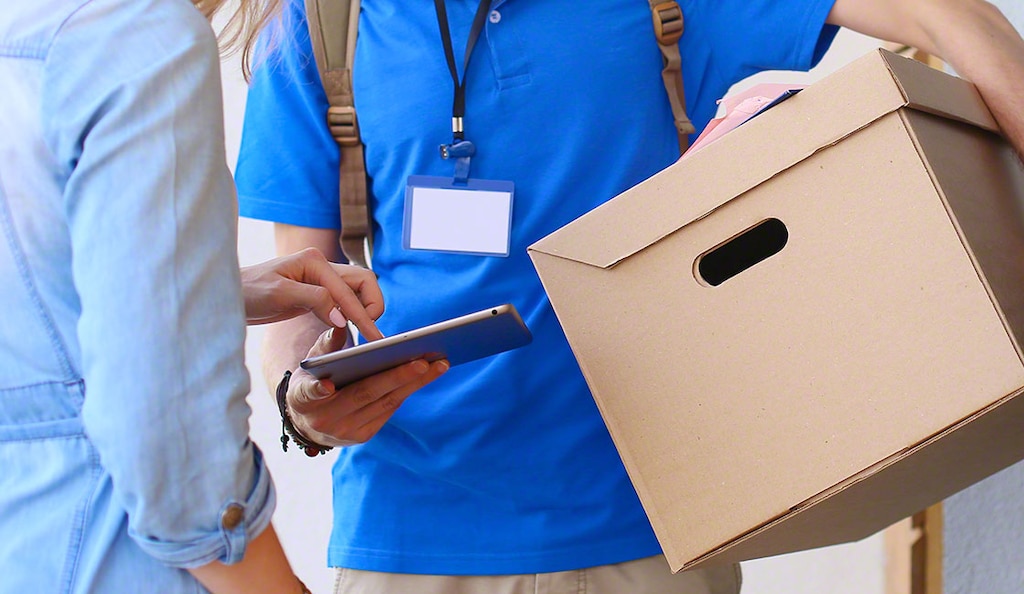
Small data: smaller, but just as efficient
While big data is characterised by the mass processing of information, small data targets specific and more manageable details to help solve specific problems and make quick decisions focused on user or customer needs. This type of information is particularly valuable for smaller enterprises or those looking for quick solutions to specific customers or problems in environments where the amount of data is not a determining factor.
In logistics, where throughput monitoring based on data generated in the facility is increasingly common, a warehouse management system (WMS) such as Easy WMS plays a key role in optimising operations. Looking to convert the large volume of data generated in your facility into valuable information? Don’t hesitate to contact us. Our team of expert consultants will be happy to advise you on the most appropriate digital solution to improve your company’s logistics operations.