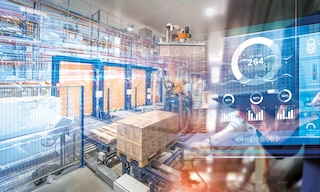
Edge AI: benefits of local artificial intelligence
Edge AI is an emerging branch of artificial intelligence currently revolutionising industry. It empowers devices to act more intelligently and autonomously, processing data within milliseconds.
What is edge AI?
Edge AI — aka AI at the edge — is the implementation of AI in an edge computing environment. It allows computations to be done close to where data are collected instead of on cloud servers or in external centres. By not needing connections to send information off-site, edge AI enables devices to make smarter decisions more quickly. Processing inputs within milliseconds, this technology provides responses and feedback in real time — almost instantaneously.
What are the benefits of using edge AI?
Employing this locally performed analysis with advanced methods such as machine learning offers the following advantages:
- Real-time analysis. Edge AI facilitates the use of high-performance computing capabilities close to the location of data sources, such as Internet-of-Things (IoT) sensors, thereby speeding up decision-making.
- Reduced latency. Data processing times are shorter since there’s no need for devices to transfer information back and forth.
- Lower bandwidth. Businesses save on cloud storage and bandwidth by processing data near the source.
- Greater security. Local processing prevents sensitive data from being exposed to potential cybercriminals by not sending it to external locations. Nevertheless, local systems are also vulnerable and susceptible to threats or attacks.
- Higher speeds. Processing capacity is more agile compared to cloud computing.
One of the biggest differences between edge AI and conventional AI is that the former doesn’t send information to a cloud system. Instead, data are analysed within connected devices operating at the edge of the network. This adds a layer of intelligence: in addition to collecting data, these devices can act through machine learning technology.
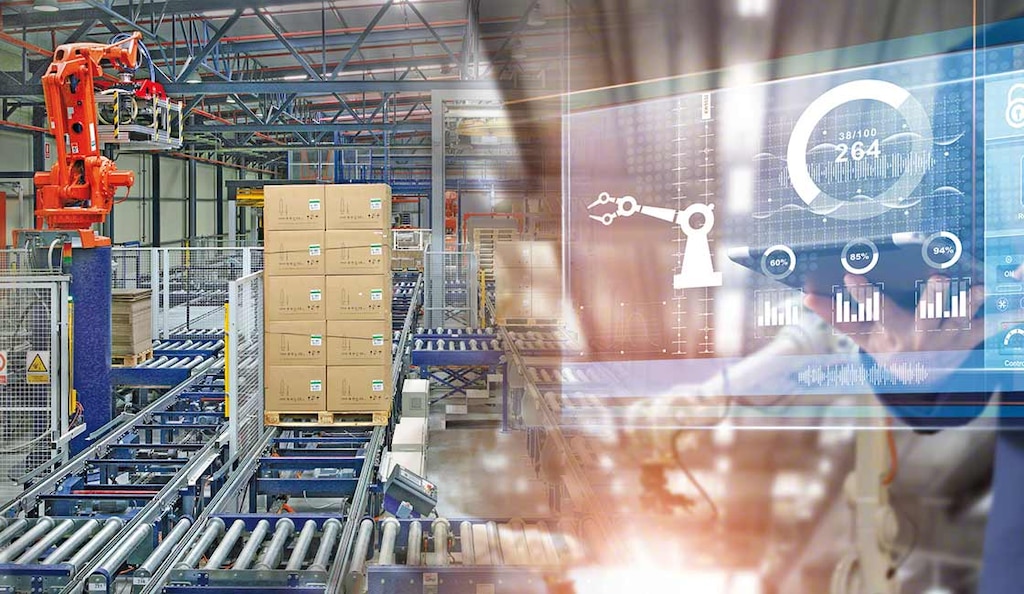
Edge AI applications in industry
Edge AI’s vast potential can be seen across numerous industrial sectors:
- Automotive. Vehicles with edge AI can make decisions like changing lanes to avoid collisions, thus improving road safety. This technology is what makes autonomous vehicles possible.
- Voice assistants. They learn to recognise voices locally to know when to activate and respond.
- Process control. Analysing data in real time makes businesses more efficient.
- Energy. Facilities such as oil and gas plants are often located in remote areas. Thanks to edge AI, they can process information independently.
- Industry and intralogistics. Robots can adapt to changes in their environment without human intervention and carry out tasks autonomously. One example is picking robots. Other applications include automating assembly lines through the use of the Industrial Internet of Things (IIoT), computer vision to inspect products for defects and predictive maintenance of equipment.
- Healthcare and patient monitoring. Treatments and diagnoses are more precise when results are analysed in real time. Additionally, tools such as heart rate and glucose monitors alert patients to any abnormalities.
- Security systems. Surveillance devices with edge intelligence can autonomously detect intruders and incidents and trigger alarms.
Likewise, edge AI computing can monitor energy consumption in industrial operations, reducing environmental impact.
Advantages and disadvantages of edge AI
To implement edge AI, local servers and devices require a certain computing capacity to execute analytical models and AI algorithms. They sometimes also have constraints in terms of memory and data storage space. If they operate on battery power, autonomy can also be considered a drawback.
To avoid these obstacles, it’s advisable to use specific architectures for low-power devices and rely on processors for AI applications (e.g., for computer vision and neural network inference).
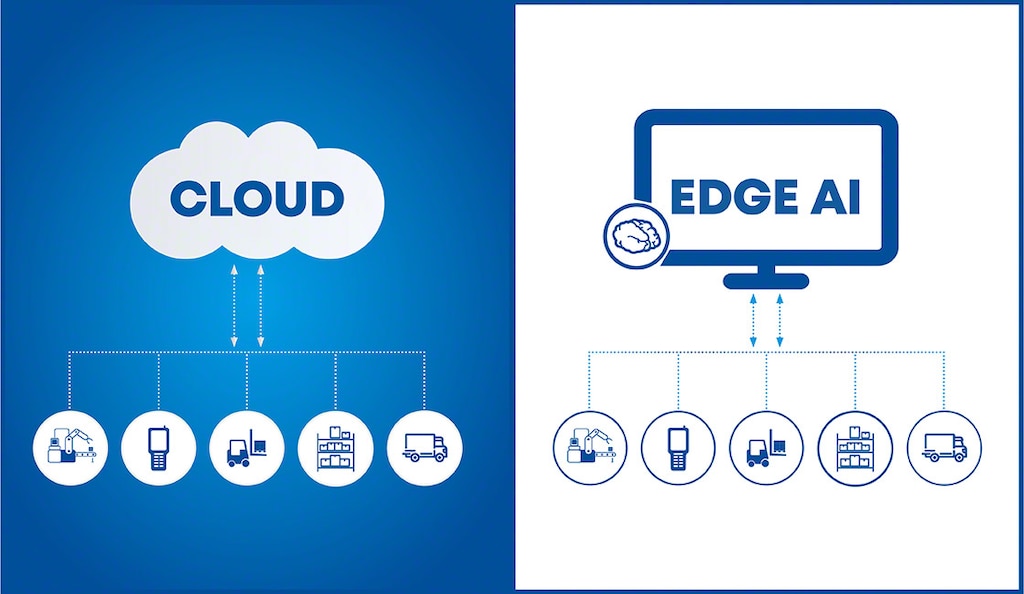
What distinguishes edge AI from cloud AI is where the algorithms are executed:
- Edge AI. Data are stored and processed on hardware peripheral to the network, such as sensors, vehicles or IoT devices. This requires less bandwidth, and latency is almost imperceptible. Edge AI can work in remote areas without connectivity. Among its disadvantages are memory limits, the need to optimise algorithms and the use of specific hardware.
- Cloud AI. Information is processed on conventional, centralised cloud servers, usually managed by a provider. Cloud AI advantages include greater capacity and computing power for managing large amounts of data. However, having to transfer data can slow down communication.
The future of logistics and AI
Edge AI is helpful in cases where information must be analysed and processed immediately. This technology is still in its early stages as we continue to see new developments in specific hardware for AI and improvements in storage and processing capacity in IoT devices.
In an increasingly interconnected world, AI plays a crucial role in logistics management. Warehouse management systems have become the best ally for rigorously controlling operations and optimising facility performance. Thinking about taking your company in this direction? Don’t hesitate to contact us. At Mecalux, we’re experts in warehouse automation and digitalisation. Our company is leading the way in AI research to expand our clients’ technological capabilities.